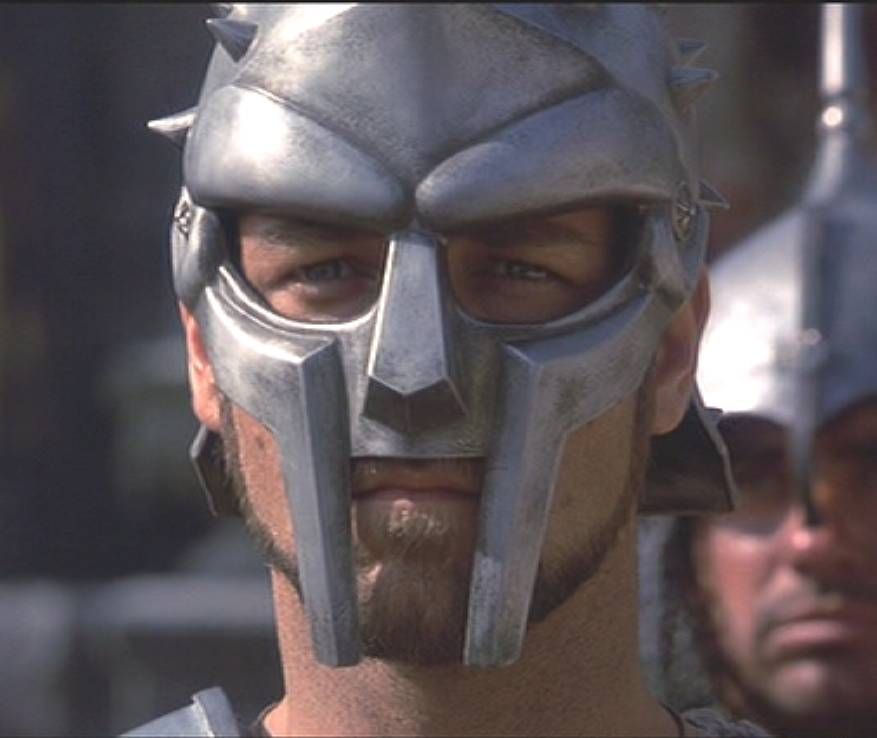
Why we should all wish we were quants.
The thing about advanced mathematics is that it can get very complicated. Once you add computer programming and random markets, it becomes very abstract. No wonder hence that so-called “quants” who develop systematic trading strategies to make money are so misunderstood. The first encounter I had with people who clearly operate on a different spectrum was in the mid nineties, when some long-haired hippie dude in flip flops walked onto the trading floor and proudly proclaimed that all of us would soon be redundant.
We had a good laugh at first, but the reality of technology taking hold of the investment world soon caught up with us. It is fair to say that none of the things we were able to do back then worked any more soon thereafter. In the first instance, that has to do with scale. Traders look to invest in opportunities that offer excess reward versus risk profiles. That probably sounds more glamorous than it is, so imagine having a shopping list of things you would like to buy, but only when they go on sale. Take the prices of Gold versus an index of Gold mining stocks and say the difference between the two has been trading in a range of 3 and 5 for quite some time. It could be a good idea to put on a trade whenever this spread gets to the outer bands of these levels, you know, because there is a pattern there.
It’s much easier to look at these things visually. Then you can also draw in lines and you can set up alerts, but the point is as a trader you are forever looking at these numbers move, only to then press a button at the right (or wrong) time. A monkey could do it really, or of course a computer. Not only that but you can program a machine to look at many more things, all the time. Whereas in the trading dark ages, success was measured in terms of the number of screens you had to look at as many things as humanly possible, the new game in town was played in nanoseconds. Today, how quickly more information can be processed by ever more powerful machines is an arms race of epic proportions.
So, where do the mathematics enter? Well, first you need to see what has worked in the past. That involves performing regressions on a lot of historical data and building a model (algorithm) to determine what you want to trade. From there you need to find the best trade entry and exit points, which entails measuring volatility and calculating the statistical probability of different outcomes. Once you get into making predictions as part of your risk management, you are into things like differential equations or nonlinear programming and far beyond basic calculus. It really does take a lot of talent to be a quant and they truly are the gladiators of modern trading. Maximus Decimus Meridius, we salute you.
Comentarios